João Paulo Papa, São Paulo State University, Brazil
Petia Radeva, Universitat de Barcelona, Spain
João Manuel R. S. Tavares, University of Porto, Portugal
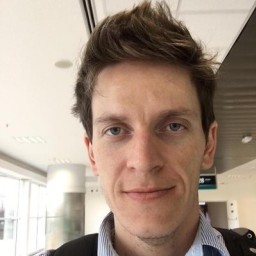
João Paulo Papa
São Paulo State University, Brazil
WEB
João P. Papa received his Ph.D. in Computer Science from the University of Campinas, SP, Brazil. During 2014-2015, he worked as a visiting scholar at Harvard University, USA. He is an associate professor at the Computer Science Department, São Paulo, State University. He is an Alexander von Humboldt Research Fellow (Germany), an IEEE Senior Member, and a Brazilian representative at the International Association of Pattern Recognition.
São Paulo State University, Brazil
WEB
João P. Papa received his Ph.D. in Computer Science from the University of Campinas, SP, Brazil. During 2014-2015, he worked as a visiting scholar at Harvard University, USA. He is an associate professor at the Computer Science Department, São Paulo, State University. He is an Alexander von Humboldt Research Fellow (Germany), an IEEE Senior Member, and a Brazilian representative at the International Association of Pattern Recognition.
ABSTRACT
Machine learning has been in the spotlight in the past decade, with astonishing results in many research domains. It is a consensus that deeply learnable features are a must-have, but improvements must be made on the classification layer. This talk discusses recent advances in the Optimum-Path Forest, a framework to design graph-based classification methods that cover supervised, unsupervised, and semi-supervised paradigms. Applications to deal with unbalanced datasets, recommender systems, and quantum computing will also be addressed.
Machine learning has been in the spotlight in the past decade, with astonishing results in many research domains. It is a consensus that deeply learnable features are a must-have, but improvements must be made on the classification layer. This talk discusses recent advances in the Optimum-Path Forest, a framework to design graph-based classification methods that cover supervised, unsupervised, and semi-supervised paradigms. Applications to deal with unbalanced datasets, recommender systems, and quantum computing will also be addressed.
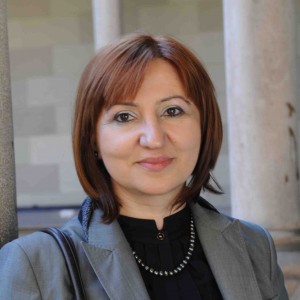
Petia Radeva
Universitat de Barcelona, Spain
WEB
Prof. Petia Radeva is a Full professor at the Universitat de Barcelona (UB), Head of the Consolidated Research Group “Computer Vision and Machine Learning” at the University of Barcelona (CVMLUB) at UB (www.cvc.uab.es) and Senior researcher in Computer Vision Center (www.cvc.uab.es). She was PI of UB in 7 European, 3 international and more than 25 national projects devoted to applying Computer Vision and Machine learning for real problems like food intake monitoring (e.g. for patients with kidney transplants and for older people). Petia Radeva is a REA-FET-OPEN vice-chair since 2015 on, and international mentor in the Wild Cards EIT program since 2017.
She is an Associate editor in Chief of Pattern Recognition journal (Q1, IP=7.196) and International Journal of Visual Communication and Image Representation (Q2, IP=3.13).
She is a Research Manager of the State Agency of Research (Agencia Estatal de Investigación, AEI) of the Ministry of Science and Innovation of Spain.
Petia Radeva belongs to the top 2% of the World ranking of scientists with the major impact in the field of TIC according to the citations indicators of the popular ranking of Stanford. Also, she was selected in the first 6% of the ranking of Spanish and foreign most cited female researchers from any field according to the Ranking of CSIC: https://lnkd.in/djx2Yz5p. Moreover, she was awarded IAPR Fellow since 2015, ICREA Academia assigned to the 30 best scientists in Catalonia for her scientific merits since 2014, received several international awards (“Aurora Pons Porrata” of CIARP, Prize “Antonio Caparrós” for the best technology transfer of UB, etc). She supervised 23 PhD students and published more than 100 SCI journal publications and 250 international chapters and proceedings, her Google scholar h-index is 50 with more than 10000 cites.
Universitat de Barcelona, Spain
WEB
Prof. Petia Radeva is a Full professor at the Universitat de Barcelona (UB), Head of the Consolidated Research Group “Computer Vision and Machine Learning” at the University of Barcelona (CVMLUB) at UB (www.cvc.uab.es) and Senior researcher in Computer Vision Center (www.cvc.uab.es). She was PI of UB in 7 European, 3 international and more than 25 national projects devoted to applying Computer Vision and Machine learning for real problems like food intake monitoring (e.g. for patients with kidney transplants and for older people). Petia Radeva is a REA-FET-OPEN vice-chair since 2015 on, and international mentor in the Wild Cards EIT program since 2017.
She is an Associate editor in Chief of Pattern Recognition journal (Q1, IP=7.196) and International Journal of Visual Communication and Image Representation (Q2, IP=3.13).
She is a Research Manager of the State Agency of Research (Agencia Estatal de Investigación, AEI) of the Ministry of Science and Innovation of Spain.
Petia Radeva belongs to the top 2% of the World ranking of scientists with the major impact in the field of TIC according to the citations indicators of the popular ranking of Stanford. Also, she was selected in the first 6% of the ranking of Spanish and foreign most cited female researchers from any field according to the Ranking of CSIC: https://lnkd.in/djx2Yz5p. Moreover, she was awarded IAPR Fellow since 2015, ICREA Academia assigned to the 30 best scientists in Catalonia for her scientific merits since 2014, received several international awards (“Aurora Pons Porrata” of CIARP, Prize “Antonio Caparrós” for the best technology transfer of UB, etc). She supervised 23 PhD students and published more than 100 SCI journal publications and 250 international chapters and proceedings, her Google scholar h-index is 50 with more than 10000 cites.
ABSTRACT
Deep Learning (DL) has made remarkable progress in tasks such as face and lip recognition or cancer detection in medical images, achieving super-human performance. However, when it comes to classifying a large number of classes, such as in fine-grained recognition, there is still much room for improvement, especially for groups of classes that are easily confused. Additionally, DL relies on greedy methods that require thousands of annotated images, which can be a time-consuming and tedious process. To address these issues, self-supervised learning offers an efficient way to leverage a large amount of non-annotated images and make DL models more robust and accurate. In this talk, we will present our work on self-supervised learning and fine-grained recognition, highlighting how this approach can help solve complex computer vision problems like food image recognition. Food classes have high variability, significant similarity between classes, and a vast number of unannotated images. By using self-supervised learning and fine-grained recognition, we demonstrate how these challenges can be overcome.
Deep Learning (DL) has made remarkable progress in tasks such as face and lip recognition or cancer detection in medical images, achieving super-human performance. However, when it comes to classifying a large number of classes, such as in fine-grained recognition, there is still much room for improvement, especially for groups of classes that are easily confused. Additionally, DL relies on greedy methods that require thousands of annotated images, which can be a time-consuming and tedious process. To address these issues, self-supervised learning offers an efficient way to leverage a large amount of non-annotated images and make DL models more robust and accurate. In this talk, we will present our work on self-supervised learning and fine-grained recognition, highlighting how this approach can help solve complex computer vision problems like food image recognition. Food classes have high variability, significant similarity between classes, and a vast number of unannotated images. By using self-supervised learning and fine-grained recognition, we demonstrate how these challenges can be overcome.
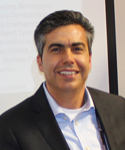
João Manuel R. S. Tavares
University of Porto, Portugal
WEB
João Manuel R. S. Tavares graduated in Mechanical Engineering at the Universidade do Porto, Portugal in 1992. He also earned his M.Sc. degree and Ph.D. degree in Electrical and Computer Engineering from the Universidade do Porto in 1995 and 2001, and attained his Habilitation in Mechanical Engineering in 2015 from the same University. He is a senior researcher at the Instituto de Ciência e Inovação em Engenharia Mecânica e Engenharia Industrial (INEGI) and Full Professor at the Department of Mechanical Engineering (DEMec) of the Faculdade de Engenharia da Universidade do Porto (FEUP).
João Tavares is co-editor of more than 80 books, co-author of more than 50 book chapters, 650 articles in international and national journals and conferences, and 3 international and 3 national patents. He has been a committee member of several international and national journals and conferences, is co-founder and co-editor of the book series “Lecture Notes in Computational Vision and Biomechanics” published by Springer, founder and Editor-in-Chief of the journal “Computer Methods in Biomechanics and Biomedical Engineering: Imaging & Visualization” published by Taylor & Francis, Editor-in-Chief of the journal “Computer Methods in Biomechanics and Biomedical Engineering” published by Taylor & Francis, and co-founder and co-chair of the international conference series: International Symposium on Computational Modeling of Objects Presented in Images, ECCOMAS Thematic Conference on Computational Vision and Medical Image Processing, International Conference on Computational and Experimental Biomedical Sciences and International Conference on Biodental Engineering. Additionally, he has been (co-)supervisor of several MSc and PhD thesis and supervisor of several post-doc projects, and has participated in many scientific projects both as researcher and as scientific coordinator.
His main research areas include computational vision, medical imaging, computational mechanics, scientific visualization, human-computer interaction and new product development.
(More information can be found at: https://www.fe.up.pt/~tavares)
University of Porto, Portugal
WEB
João Manuel R. S. Tavares graduated in Mechanical Engineering at the Universidade do Porto, Portugal in 1992. He also earned his M.Sc. degree and Ph.D. degree in Electrical and Computer Engineering from the Universidade do Porto in 1995 and 2001, and attained his Habilitation in Mechanical Engineering in 2015 from the same University. He is a senior researcher at the Instituto de Ciência e Inovação em Engenharia Mecânica e Engenharia Industrial (INEGI) and Full Professor at the Department of Mechanical Engineering (DEMec) of the Faculdade de Engenharia da Universidade do Porto (FEUP).
João Tavares is co-editor of more than 80 books, co-author of more than 50 book chapters, 650 articles in international and national journals and conferences, and 3 international and 3 national patents. He has been a committee member of several international and national journals and conferences, is co-founder and co-editor of the book series “Lecture Notes in Computational Vision and Biomechanics” published by Springer, founder and Editor-in-Chief of the journal “Computer Methods in Biomechanics and Biomedical Engineering: Imaging & Visualization” published by Taylor & Francis, Editor-in-Chief of the journal “Computer Methods in Biomechanics and Biomedical Engineering” published by Taylor & Francis, and co-founder and co-chair of the international conference series: International Symposium on Computational Modeling of Objects Presented in Images, ECCOMAS Thematic Conference on Computational Vision and Medical Image Processing, International Conference on Computational and Experimental Biomedical Sciences and International Conference on Biodental Engineering. Additionally, he has been (co-)supervisor of several MSc and PhD thesis and supervisor of several post-doc projects, and has participated in many scientific projects both as researcher and as scientific coordinator.
His main research areas include computational vision, medical imaging, computational mechanics, scientific visualization, human-computer interaction and new product development.
(More information can be found at: https://www.fe.up.pt/~tavares)
ABSTRACT
The segmentation of objects in images by computational approaches is very challenging, and it can be tackled using, for example, thresholding, deformable models built on statistical, geometrical or physical principles, registration, and/or machine learning-based approaches. Examples of current applications of object segmentation include the identification of regions and structures of Engineering and the delineation of lesions and tissues in Biomedicine.
In this lecture, techniques we have developed to segment images acquired using different imaging modalities will be described, and their use in engineering and medicine applications will be discussed.
The segmentation of objects in images by computational approaches is very challenging, and it can be tackled using, for example, thresholding, deformable models built on statistical, geometrical or physical principles, registration, and/or machine learning-based approaches. Examples of current applications of object segmentation include the identification of regions and structures of Engineering and the delineation of lesions and tissues in Biomedicine.
In this lecture, techniques we have developed to segment images acquired using different imaging modalities will be described, and their use in engineering and medicine applications will be discussed.